In the dynamic arena of today’s business world, the defining element steering industries globally is data. As this silent yet potent player gains inevitable prominence, the tool that effectively manipulates its relevance is data analytics. The following discourse delves into the expanding ecosystem of data analytics in a business magnifier. It illustrations the nuances from understanding what the concept embodies and its overwhelming benefits to how it can be effectively implemented in businesses. Furthermore, indispensability of data analytics in shaping strategies and decision making are discussed, finally addressing the sensitivities revolving around the ethics and privacy considerations of data analytics.
Understanding Data Analytics
Understanding Data Analytics
Data analytics refers to the science of examining raw data with the purpose of drawing conclusions about the information it contains. This complex process involves several stages including data collection, cleaning, modeling, interpretation, and finally, decision-making. Businesses can use this intricate structure of analysis to perceive trends, patterns, and insights that would otherwise remain concealed within the vast quantities of data they generate.
Types of Data Analytics
Among the various types of data analytics, four main categories are descriptive, diagnostic, predictive, and prescriptive analytics.
Descriptive analytics use data aggregation and mining techniques to provide insight into the past and answer: “What has happened?” Diagnostic analytics delve deeper to understand why something happened.
Predictive analytics, as the term suggests, uses statistical models and forecasting techniques to understand the future. They ask: “What could happen in the future?”
Prescriptive analytics go a step further, suggesting various course of actions on what action to take. They answer: “What should we do if these trends continue?”
Data Analytics Tools
The tools encompassed in data analytics range from artificial intelligence to big data, and each tool has its unique role and function. Predictive and prescriptive analytics use statistical algorithms and machine learning techniques to predict future outcomes based on historical data.
Machine Learning (ML), an application of artificial intelligence (AI), provides systems the ability to learn and improve from experience without being explicitly programmed. These technologies can identify patterns and trends that would be too complex for humans to notice, enabling businesses to make more informed decisions.
Key Terminologies
There are a number of key terminology associated with data analytics. Some of the common terms include data mining, which is the process of examining large databases to generate new information; and big data, which refers to extremely large sets of data that can be analyzed computationally to reveal patterns, trends, and associations.
The Evolution of Analytics in Contemporary Business
Data analytics have tirelessly evolved, marking their relevance in the business realm. Earlier stages of business development witnessed the utilization of rudimentary analytics tools, solely purposed for accumulating transaction summary data. As technology underwent necessary advancements, businesses adapted by curbing their reliance on these methods and incorporating more complex techniques designed to analyze intricate data sets, predict emergent trends, procure actionable insights, and most importantly, facilitate distinctly informed business decisions.
Throughout its existence, data has remained a vital cog in the wheel of business operations. Still, it is the dramatic evolution of data analytics technology that has marked a paradigm shift in the way businesses strategize and function. In the midst of a capricious environment, data analytics stands as a beacon of lucidity, offering fact-based decision making to navigate towards sustained growth and profitability.
Today, data analytics are not simply appreciated – they are essential. Businesses utilise them not only to reflect on past performance but also to foresee future trends and their potential impact. This assists businesses in reaching informed decisions, identifying market trends, pointing out business issues and opportunities, refining business practices, cutting costs, and enhancing their productivity and profitability. Essentially, data analytics play a pivotal role in shaping a company’s strategic direction by enhancing decision-making processes. Thus, in the hands of decision-makers, data analytics emerge as a powerful tool capable of fostering business growth and boosting innovation.
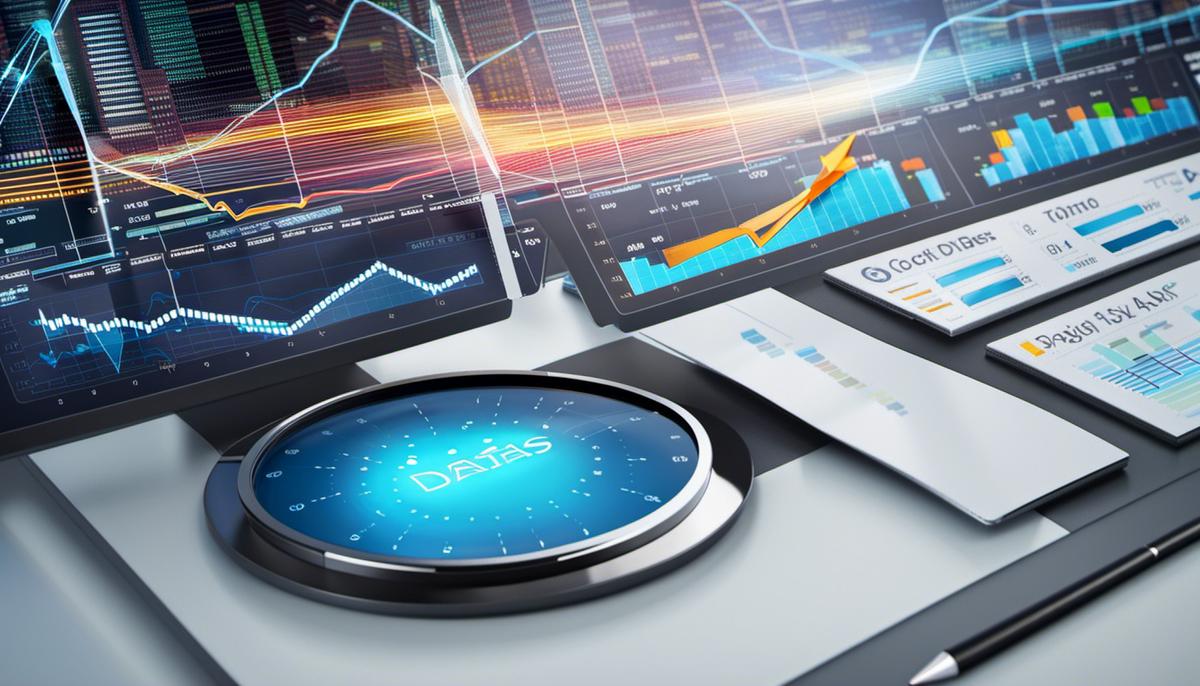
Benefits of Data Analytics in Business
Untapping the Potential of Data Analytics in Business Decision-Making
Illuminating the biggest advantage of integrating data analytics into business is its ability to usher businesses into a realm dominated by informed decision-making processes. No longer do businesses have to rely solely on personal intuition or past experiences. They can now lean on real, data-driven insights for making crucial strategic decisions. Data analytics offer insights into market trends, customer tendencies, and product performance, thereby enabling businesses to take proactive, informed decisions tailor-made for success. A profound example of the power of data analytics is reflected by Netflix. By employing data analytics to understand viewer preferences, it has been able to deliver hit series like House of Cards and Stranger Things, clearly indicating the influential role data analytics has to play within decision-making processes.
Data Analytics Enhance Operational Efficiency
Data analytics can also enhance operational efficiency in businesses. By analyzing data, companies can identify bottlenecks in their operations, find areas for process improvement, and optimize their resources to increase productivity. One example is the use of data analytics in the logistics industry. Companies like UPS use data analytics to optimize delivery routes, improve fuel efficiency, and reduce delivery times – resulting in significant cost savings and improved customer service.
Increasing Revenue with Data Analytics
Besides aiding decision-making and improving efficiency, data analytics can also drive revenue growth. With information about customer preferences and buying behavior, companies can identify opportunities for upselling or cross-selling, design targeted marketing campaigns, or develop new products that meet the needs of their customers. A case in point is Amazon, which applies data analytics to recommend products based on customers’ previous purchases, thus potentially increasing sales.
Data Analytics for Competitive Advantage
In today’s highly competitive business environment, gaining an edge over competitors is crucial. Data analytics can provide this advantage by offering in-depth knowledge about market trends, customer behavior, and competitors’ activities. By leveraging this information, businesses can anticipate market shifts, adapt quickly, and stay ahead of the competition. For instance, Spotify uses data analytics to provide personalized playlists, thus setting it apart from other music streaming services.
Transforming Industries through Data Analytics
Across industries, we see the transformative power of data analytics. In Healthcare, predictive analytics helps in forecasting disease outbreaks, improving patient care, and designing effective treatment plans. The finance industry uses data analytics for risk assessment, fraud detection, and customer segmentation. Meanwhile, retail businesses use it for inventory management, customer segmentation, and price optimization.
In a nutshell, data analytics has evolved into an indispensable instrument that businesses from all sectors and sizes can utilize to enhance decision-making processes, optimize efficiency, boost revenue, obtain a competitive advantage, and ultimately, fuel growth and prosperity.
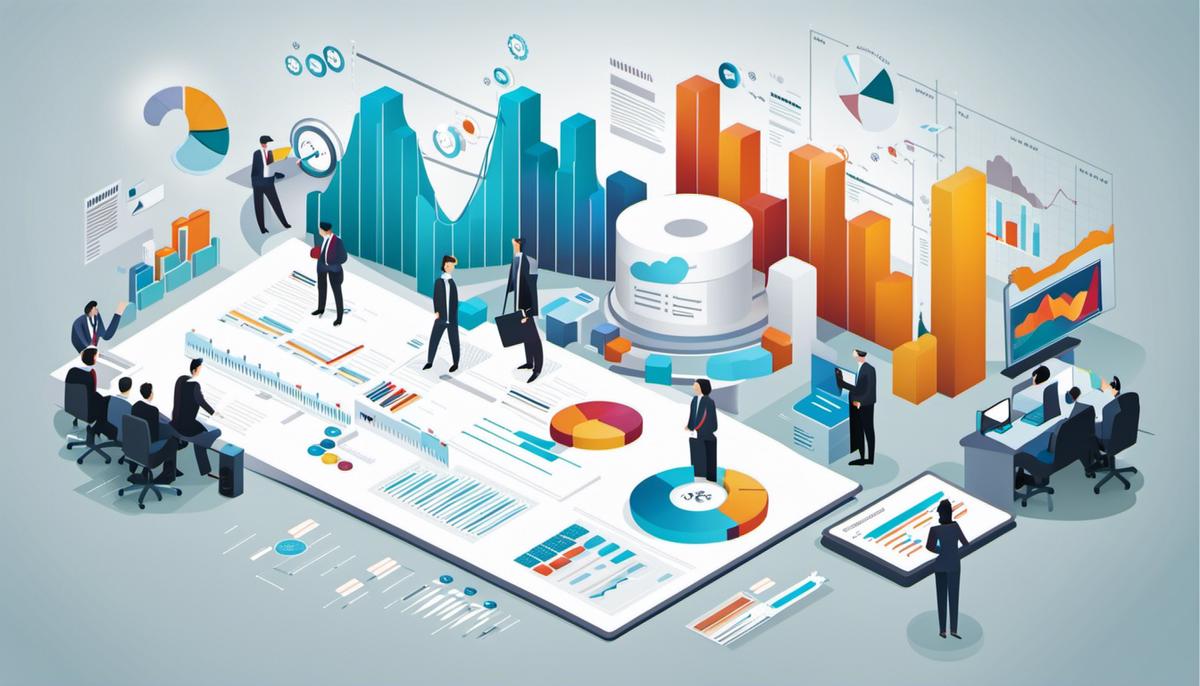
Implementation of Data Analytics
Kickstarting Data Analytics with Data Collection
For a business intending to implement data analytics, the journey starts with data collection. This crucial step encompasses the accumulation of data related to the business’s operations and strategic goals. Data can be gathered from a variety of sources like customer interactions, ecommerce transactions, social media activity, surveys, and specialized tools like web analytics. The quality and diversity of the collected data carry substantial weight in the subsequent stages and as such, ensuring data validation and variety in data sources is vital.
Data Processing: Transforming Raw Data into Understandable Formats
Following the collection of data, data processing translates raw data into a format that can be easily analyzed. This stage can involve a range of operations, depending on the needs of the business, such as classifications, sorting, summarizing, aggregation, and tabulation. Data processing is vital as it refines the data and makes it convenient for analysis, thus enabling businesses to extract targeted and relevant insights.
Data Cleaning: Ensuring Data Quality
After data processing, it is crucial to perform data cleaning. Also known as data cleansing or scrubbing, this process involves detecting, correcting, or removing corrupt, inaccurate, irrelevant, or duplicate data. Cleaning enhances the data’s quality and reliability and ensures that any subsequent analysis and interpretation are based on accurate and trustworthy data.
Data Analysis and Interpretation: Extracting Valuable Insights
Data analysis is the core stage of data analytics. This stage involves using statistical techniques and algorithms to discover patterns, correlations, and trends within the processed and cleaned data. Advanced technologies like machine learning and artificial intelligence can be leveraged at this stage to analyze complex datasets and derive deeper insights.
The analysis is followed by interpretation, where the discovered patterns and trends are contextualized and related back to the business objectives. This can involve transforming complex data into understandable reports, charts, or visualizations. This leads to actionable insights that can inform decision-making, optimize operations, and drive business growth.
Role of Data Analysts and Data Scientists
The above stages highlight the significant roles of data analysts and data scientists in data analytics implementation. These professionals carry out the technical processes, from data collection to interpretation. They possess deep knowledge of statistical techniques, data mining methods, and data visualization tools.
Data analysts focus on examining large datasets to identify trends, develop charts, and create visual presentations to help businesses make more strategic decisions. On the other hand, data scientists use their expertise in both technology and social science to find trends and manage data. They often use predictive modeling to create real-time and dynamic analytic models that can drive business growth.
Challenges and Solutions in Implementation
Implementing data analytics in a business context can come with challenges. These may include data privacy concerns, data integration issues, lack of skilled personnel, and financial constraints. To overcome these, businesses can adopt privacy-preserving computation techniques, use data integration tools, invest in capacity building, and use cost-effective data analytics platforms.
Final Thoughts
Applying data analytics is paramount when it comes to fueling informed and strategic business choices. This undertaking, however, can be rather complex as it necessitates the amalgamation of the appropriate data, sophisticated technology, and talented personnel.
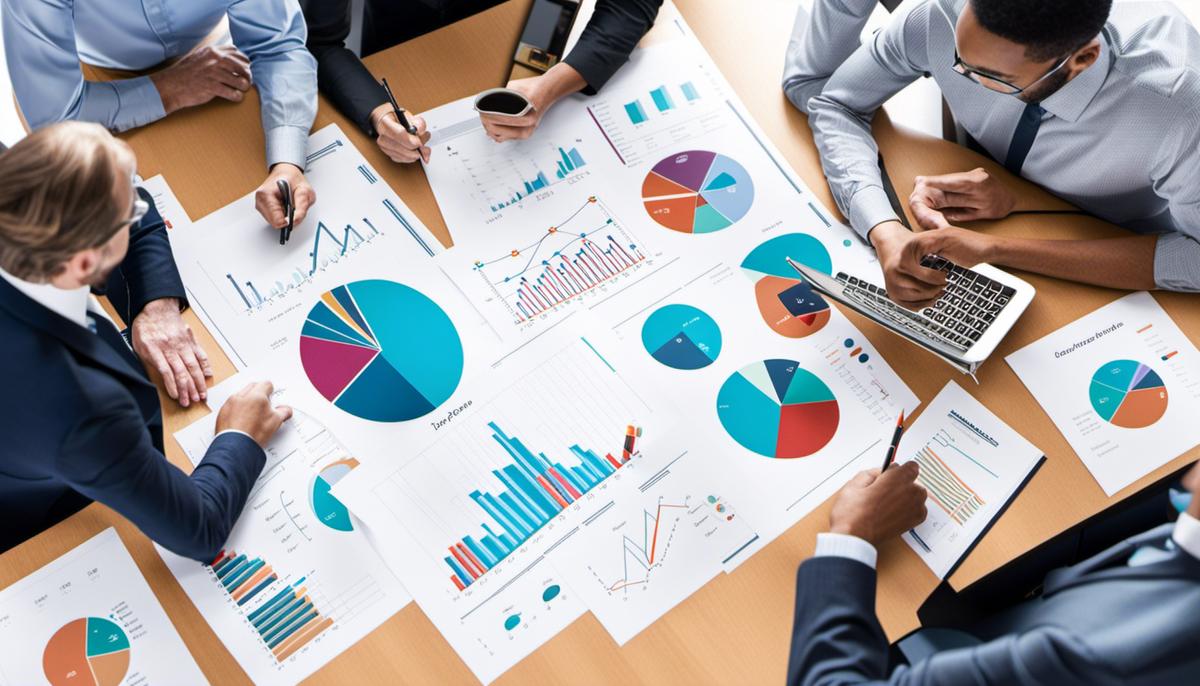
Role of Data Analytics in Strategy and Decision Making
The Significant Impact of Data Analytics on Business Strategies and Decision-Making
The use of data analytics has become a cornerstone in devising business strategies and making important decisions. Organizations from varying industries use data analytics as a means to forecast trends, gain insight into customer tendencies, project future scenarios, and mitigate potential risks. Analytics tools act as catalysts, promoting data-driven decision-making. This, in turn, not only fortifies competitiveness but also prepares companies for future challenges in an ever-changing business landscape.
Predictive Capabilities of Data Analytics
One of the most important roles of data analytics in a business context is its predictive capabilities. Through machine learning algorithms and statistical models, data analytics can predict potential future outcomes based on historical data. For example, a retailer could use predictive analytics to determine which products are likely to sell well in the coming season, allowing them to make informed decisions about stock levels. Likewise, an insurance company could use predictive analytics to calculate the probability of a claim, which could help them to set their premiums accurately.
Data Analytics for Identifying Trends
Data analytics also aids in identifying trends that can shape business strategy. By analyzing large amounts of data from various sources, companies can identify emerging patterns and trends. An e-commerce business could use data analytics to understand the shopping patterns and preferences of their customers, and then tailor their product offerings based on these insights. Similarly, a financial institution can identify patterns of fraudulent transactions and take steps to prevent such activities from occurring in the future.
Understanding Customer Behavior through Data Analytics
Understanding customer behavior is crucial for any business, and data analytics serve as a powerful tool in achieving this. By analyzing data from customer interactions across various channels, businesses can build detailed customer profiles and understand their preferences and purchasing habits. This means businesses can create personalized marketing campaigns, develop products that cater to customers’ wants and needs, and improve customer service, leading to increased customer loyalty and revenue.
Forecasting Future Scenarios with Data Analytics
Data analytics plays a powerful role in forecasting future scenarios. By analyzing current and historical data, businesses can predict future sales, market trends, and customer behavior. This allows them to plan their strategies effectively to attain future growth and success. For example, businesses can use data analytics to forecast future sales, enabling them to manage inventory more effectively and make strategic pricing decisions.
Mitigating Risks through Data Analytics
Risk mitigation is another significant area where data analytics finds application in business. By identifying trends and predicting potential future outcomes, businesses can reduce both operational and strategic risks. Companies can use data analytics to detect anomalies, predict equipment failures, and detect potential fraud or cybersecurity threats. This ability to foresee and mitigate risks can drastically reduce costs and prevent disastrous consequences.
In essence, data analytics play a pivotal role in business strategy formulation and decision-making, acting as a transformative agent. By identifying trends, understanding customer behavior, predicting future occurrences, and providing risk mitigation avenues, data analytics equips organizations with the crucial information they need to make strategic, well-informed decisions.
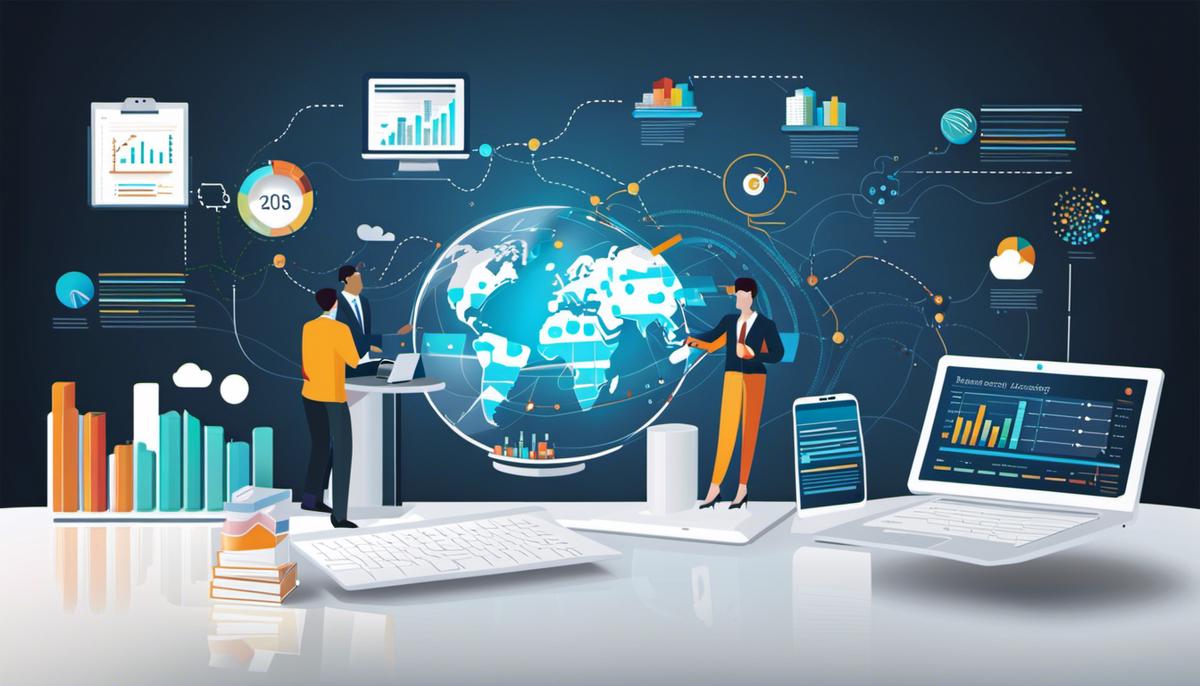
Data Analytics Ethics and Privacy Considerations
Delving into the Ethics and Privacy Aspects of Data Analytics
The ethical dimensions of data analytics, a critical facet of this field, govern its use and application within a business context. Grasping and adhering to the ethical parameters, laws, regulations, and societal expectations concerning data use, storage, security, and sharing is a responsibility for all businesses. Parallelly, considerations of privacy are of equal importance, ensuring protection of personal details, confidentiality of sensitive information, and upholding the rights of individuals with regards to their own data.
Legal Regulations in Data Analytics
There are numerous legal regulations globally that govern data analytics to maintain ethical practices and safeguard privacy. These include laws such as the General Data Protection Regulation (GDPR) in the European Union, the California Consumer Privacy Act (CCPA) in the United States, and other similar legislations in different countries. These laws mandate companies to adopt specific data management practices, including the duty to inform consumers about how their data will be used, providing consumers with the right to opt-out of data collection or to delete their personal data, and obligation to safeguard consumer data against security breaches.
Ethical Dilemmas in Data Analytics
Despite the existence of such laws, businesses often face several ethical dilemmas in their use of data analytics. For example, the question of what data should be collected and how much of it can sometimes be ambiguous. The manner in which data is collected can also pose ethical questions, particularly when users are unaware of the extent or purpose of the data collection. Additionally, there are also concerns about bias in data analytics, as algorithms used in this process may inadvertently discriminate against certain groups if not properly checked and managed.
Privacy Concerns in Data Analytics
Data analytics can inadvertently infringe upon individual privacy through the collection, processing, and storage of personal data. This can happen especially in the case of Big Data, where vast amounts of data are collected from numerous sources. Even with anonymized data, techniques such as data de-anonymization can pose a threat to privacy, as they can potentially re-identify individuals from anonymized data sets.
Addressing Challenges in Data Analytics Ethics and Privacy
To address the challenges associated with ethics and privacy in data analytics, businesses should adopt a comprehensive approach that involves clear regulation, transparency, and accountability. Firstly, businesses must fully understand the laws and regulations pertaining to data privacy in their respective jurisdictions and ensure compliance with these laws.
Moreover, they should maintain transparency in their data collection and analysis practices, informing users about what data is being collected, how it is used, and what measures are in place to protect their privacy. It’s also crucial to reinforce accountability in the organization, implying that any misuse of data or breach of privacy should be promptly addressed and the responsible parties held to account. Plus, creating a balance between the value obtained from data analytics and privacy concerns is critical for ethical business practices.
Moreover, employing robust data security measures can help safeguard against breaches, while periodic audits can help ensure ongoing compliance and rectify any potential issues. Implementing privacy-enhancing technologies, such as differential privacy, can also help make data analytics less invasive.
Considering ethics and privacy from the start, when designing data analytics processes, can help identify and mitigate potential issues before they occur. This includes considering who the data will impact, how it will impact them, what potential harms may arise, and how to prevent those harms.
Finally, employee training and awareness are essential to ensure everyone in the organization understands their role in maintaining data ethics and privacy. By promoting a culture of responsible data handling and respect for privacy, businesses can ensure ethical and legal data analytics practices.
In summary, to effectively manage data analytics ethics and privacy, businesses need a combination of legal adherence, ethical awareness, transparency, accountability, security measures, technological tools, thoughtful design, and ongoing vigilance.
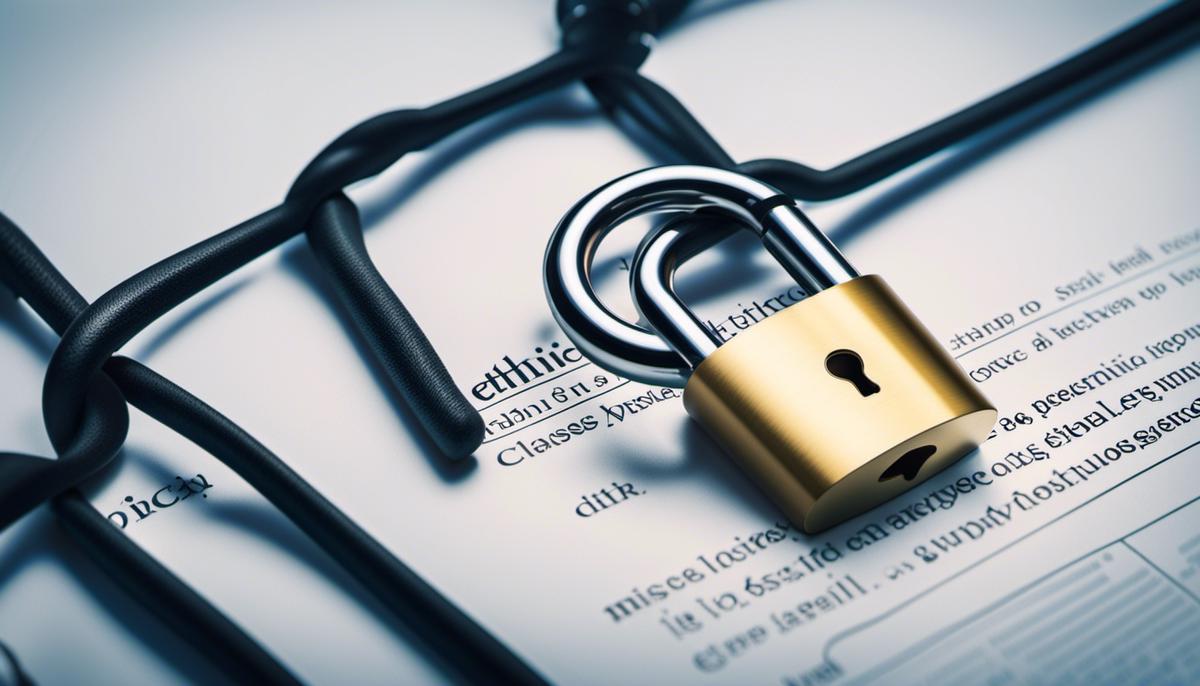
As we advance, it becomes evident that data analytics stands as an indispensable ally in the robust architecture of corporate operations. Through understanding its fundamentals and employment, businesses can unlock doors to unprecedented efficiencies and insights. Spanning from granular decision-making facets to aligning with privacy and ethical mandates, data analytics has profoundly resonated in virtually every business strategy. Imbued with profound capabilities of predictive intelligence and risk mitigation, it empowers businesses to navigate through the uncertainties of the market, thereby establishing a formidable competitive edge in their respective industries.